Podcast Language and Engagement

August 03, 2021 Published by Sravana Reddy and Rosie Jones
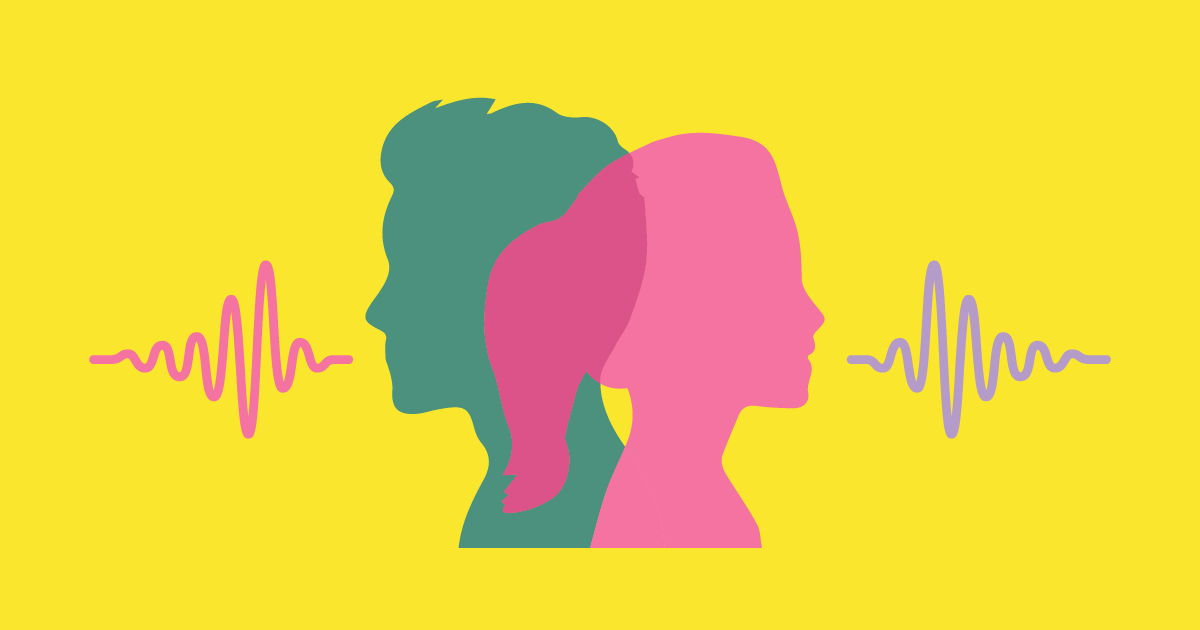
What makes a particular podcast broadly engaging? As a media form, podcasting is new enough that such questions are only beginning to be understood. Websites exist with advice on podcast production but there has been little quantitative research into how aspects of language usage contribute to listener engagement. In our ACL 2021 paper, we investigate how various factors – vocabulary diversity, distinctiveness, emotion, and syntax, among others – correlate with engagement, based on analysis of the podcast creators’ written descriptions and transcripts of the audio.
The data
In 2020, we released Spotify English-Language Podcast Dataset, a collection of over 100,000 English-language podcast episodes. Each episode belongs to a parent “show”. The dataset contains automatically generated transcripts of the audio, as well as other textual information such as titles and descriptions. We also obtained streaming numbers for the episodes in the dataset from Spotify, aggregated from the date of the episode’s publication on the platform until December 2020. We use stream rate as the engagement metric, defined as the proportion of the show’s first-time listeners who stream at least five minutes of the episode. We filter out all episodes that are shorter than ten minutes and fewer than a threshold number of total streams. To control for duration effects in the analysis of transcripts, we truncate transcripts at ten minutes. The resulting dataset has about 5000 episodes.
Which linguistic features correlate with engagement?
Through a combination of reading podcast advice blogs, previous research on correlating linguistic features with consumption metrics in other media like books and tweets, and intuition, we devised a set of interpretable, automatically measurable features of the titles, descriptions, and transcripts. These are features like the proportion of swear words or the reading grade level.
Much of the popular advice of language usage is validated by the data. Compared to low engagement episodes, high engagement podcast episodes tend to have longer and more relevant descriptions, use diverse vocabularies (as measured by word entropy and reading grade level), contain more positive emotions and fewer negative emotions, more conversations and personal narratives (as measured by the prevalence of first and second person pronouns compared to third), and fewer words associated with swearing.
On the other hand, some of the correlations are surprising. High engagement podcast episodes use language more like the average podcast creator, as measured by the cross entropy of the episode under a language model trained on the rest of the dataset, which contradicts the general advice to create a distinctive “voice.” They are also associated with faster speech rates (number of words per second) than low engagement episodes.
We gained insights about aspects that are not found in advice blogs as well. For example, it turns out that high engagement podcasts are more likely to contain words associated with anticipation. They also contain more conjunctions and determiners, but fewer proper nouns and adjectives.
It must be emphasized that the stylistic associations that were observed to distinguish high and low engagement podcasts in this particular dataset are correlations with no causality established, and therefore must be interpreted with caution.
Can we predict if a podcast will be engaging from content alone?
Given the strong correlations between engagement and the linguistic features, our next question is whether these features can predict whether an episode falls into the high engagement group. We find that a simple linear classifier with the above linguistic features can predict (with over 71% accuracy) whether a podcast will be in the top 25% of podcasts by stream rate in our dataset or the bottom 25%. Since these features largely encode style rather than topics, this suggests that linguistic style is highly predictive of engagement. A richer model using BERT is even more accurate, at nearly 81%.
What’s left?
We focused on a subset of easily computable textual features inspired by popular advice to podcast creators. We hope that this work will spur more research into the relationship between engagement and other linguistic features, especially those related to speech, such as prosody, acoustic quality, and speaker identities. It will also be valuable to consider how linguistic features engage different subcommunities of listeners.
More information about the methodology, experiments and related work can be found in our paper:
Modeling Language Usage and Listener Engagement in Podcasts
Sravana Reddy, Mariya Lazarova, Yongze Yu, Rosie Jones