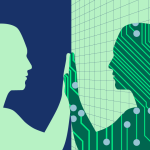
Answering counterfactual “what-if” questions in a trustworthy and efficient manner
April 20, 2023 12:56 pmAnswering causal questions with machine learning algorithms is a challenging yet critical task.
Answering causal questions with machine learning algorithms is a challenging yet critical task.
Quantifying cause and effect relationships is of fundamental importance in many fields, from medicine to economics. The gold standard solution to this problem is to conduct randomised controlled trials, or A/B tests.
Understanding cause and effect relationships in Spotify data to inform decision-making is crucial for best serving Spotify’s users and the company.
There are many A/B tests we might like to run, but which are too technically challenging, risky in terms of user impact or even impossible to perform. For instance, in the classic example of whether smoking causes lung cancer, forcing a randomly selected group of people to smoke is unethical if we believe it might damage their health. In the context of technology companies, if we want to understand if app crashes cause users to churn, we would have to randomly select a subgroup of users and crash their apps on purpose – not something we would want to consider given we do not want to break their trust....