Researching how less-streamed podcasts can reach their potential

One question we spend a lot of time thinking about at Spotify is how to help creators build larger audiences, so they have a larger reach and an easier time making a living from their creative work. In the realm of podcasts, from the mainstream to the niche, each podcast has some potential unreached audience and could benefit from strategic audience matching. That being said, mainstream podcasts do not need as much help building an audience – a large fraction of people are interested in giving it a try, so recommending them even to randomly-selected users helps them grow. However, a high-quality but more focused podcast may be of interest to a smaller group of people, so recommending it to randomly-selected users would not work nearly as well. In this research paper, we refer to such podcasts, which are typically the most difficult to recommend effectively, as “underserved” podcasts.
Underserved podcasts require more strategic recommendations to match them to users who are more likely to be interested in them. By having conducted research on this topic we found that it is important to identify these podcasts and focus attention on better audience matching for them. The research tackles this problem from three angles:
In order to define and identify underserved podcasts more clearly, we explored characteristics of less-streamed podcasts through a large-scale online randomized study of 5M recommendations.
We carried out observational studies on the listening behaviors of podcast users on Spotify, and showed the potential for increasing the audiences of these underserved podcasts.
In an offline study, we found that semantic information improves discovery not only for underserved podcasts, but also overall.
Identification of underserved podcasts
Spotify hosts about 3.2 million podcasts on a wide variety of topics. Podcasters often find ways of promoting their own work: Some podcasts have marketing budgets and are able to advertise in various ways, and sometimes users find podcasts through word of mouth or social media. In order to help users navigate this large space, we use machine learning systems to recommend podcasts to users we think may be interested in them. However, for podcasts with small audiences and low consumption, it is harder for us to learn which types of users are interested in them.
Generally, an “underserved” podcast could be thought of as any show which has a hard time building an audience “naturally,” and would benefit the most from targeted recommendation. To formally define underserved podcasts, we first established a baseline by recommending podcasts to users at random and measuring how often they are streamed on average. We then looked for podcast characteristics that would be indicative of whether podcasts will be streamed by random users less often than the baseline. The main idea here is that if randomly-selected users are less likely to stream a given podcast compared to other podcasts, then that podcast needs more strategic audience matching.
Characteristics of Underserved Podcasts
The table below shows how different subsets of podcasts fared relative to the global average stream rate. The first column identifies a particular group of podcasts, the second column indicates what fraction of the recommendations in our study fell within that group, and the third column reports how much streaming that group received from randomly selected users, relative to the average (mean) taken across all the podcasts in our study.
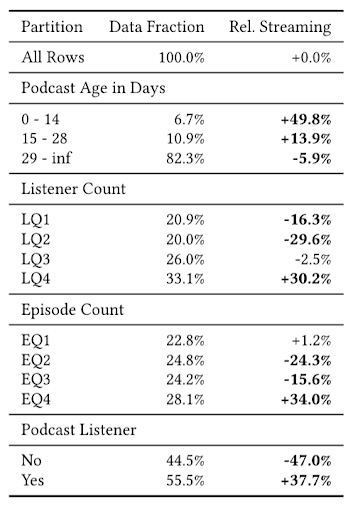
The recommendations are broken down by podcast age, listener count quantiles (LQ1– LQ4, where group LQ1 had the fewest listeners and LQ4 had the most), episode count quantiles (EQ1 – EQ4, where again EQ1 had the fewest episodes), and whether the user was an existing podcast listener. Numbers in bold are statistically significant. We found the following trends from this data:
Recency factor: a podcast less than 14 days old performs better.
In general, podcasts that already have more listeners do better. Users could not see the listener count to influence their decision, so they were not simply responding to a podcast’s popularity. This suggests that some podcasts have more general appeal, perhaps explaining why they already had higher listener counts.
Podcasts with the most episodes (EQ4) do better. Users could see the episodes for a podcast, and they seemed to prefer podcasts that already had more content. We will discuss the interaction between this and podcast age below.
We further looked at the relative streaming rates for each combination of podcast age and listener count. Among podcasts more than 28 days old, the podcasts that got the most streams had the most existing listeners.
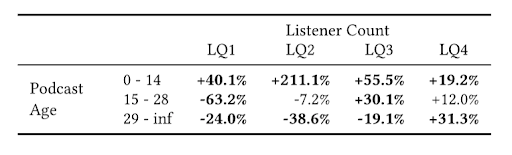
Looking at the relative streaming rate for each combination of podcast age and episode count, among podcasts more than 28 days old, the larger the number of episodes the more likely a user was to stream the podcast. However, only podcasts in EQ4 – with the highest numbers of episodes – were more likely than average to be streamed by a randomly-selected user.
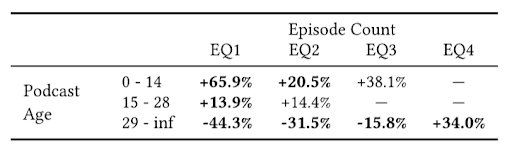
Based on this randomized study, we identified podcast characteristics that are related with more streaming:
Young podcasts, less than 28 days old and especially less than 14 days old, are much more likely to be streamed.
Among podcasts more than 28 days old, the larger the number of listeners or episodes the more likely a randomly-selected user was to stream the podcast.
We formally identify underserved podcasts as being more than 28 days old and having either “fewer” listeners or “fewer” episodes.
Underserved Podcasts’ Growth Potential
We next looked at whether underserved podcasts could grow larger audiences. This is important to examine in order to rule out the possibility that users are already good at finding the podcasts they would like, and that strategic recommendation might not be much help. If underserved podcasts do grow over time, this helps to justify recommending more strategically, accelerating the audience building process.
We found that underserved podcasts are, in fact, likely to grow over time. We further found that older podcast episodes are still of interest to some listeners. This helped us conclude that well-targeted recommendations may help these podcasts find more listeners faster. The next question was: how can we successfully recommend these underserved podcasts?
Better podcast-audience matching
Our approach to matching underserved podcasts with new listeners is to leverage content understanding tools, and particularly Knowledge Graphs, to find users who already listen to podcasts that are similar to a given underserved podcast. We wanted to test the hypothesis that semantic similarity between a recommended podcast and those from a user's listening history can improve podcast-audience matching.
We used semantic information by means of a podcast knowledge graph (KG) to do this matching. KG’s are a way to organize information and relate different pieces of information, called entities, to each other. Examples of entities are topics discussed in a podcast, information about podcast’s guests and hosts, and so on. For example, in a KG we might connect a particular guest to all of the podcasts in which they have appeared. We built a large podcast KG to use for these recommendations. Some statistics about our podcast KG entities are reported in the table below. We then applied a machine learning technique called embedding on the KG to create vector representations of the podcasts. This allowed us to measure how similar a candidate podcast for recommendation is to the user’s favorite shows. We then recommend podcasts closest to our estimate of the user’s taste, based on the podcasts they already listen to.
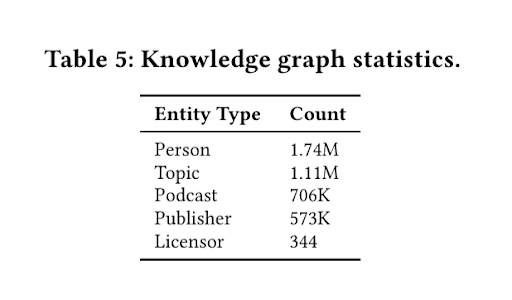
We compared the performance of the KG features to some baseline features. One baseline model included only consumption features and user demographic features. Our second baseline model also included collaborative filtering (CF) features. We tested such features against adding KG features in both logistic regression as well as Generalized Additive Models (GAM). We find that adding KG features significantly improves model performance compared to baseline models. Our results show that semantic information helps underserved podcast discovery, suggesting that relevance to users’ listening history does indeed help to predict user interest in underserved podcasts.
Logistic Regression Results
The table below reports the Average Precision performance of our logistic regression models. Average Precision measures what fraction of the podcasts recommended by the model were actually streamed. The Basic column is our baseline model, which uses some basic demographic and historical consumption information about users. The columns with the prefix +KG show how two different models trained on slightly different knowledge graphs performed, relative to the Basic model. The columns with the +CF prefix are a pair of baselines which use collaborative filtering features instead of knowledge graph features. Collaborative filtering provides a way to recommend to users of a given podcast based on which other podcasts other users also listen to.
As the table shows, the +KG models produced substantially more streaming than either the +CF or Basic models. They performed well for all podcasts and also specifically for underserved podcasts. The best performance overall was +KGsm on underserved podcasts.
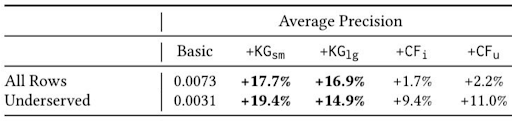
What’s next?
Our work so far has focused on a small set of professionally-produced podcasts, and we want to expand it to include all types of podcasts. We think it is important to understand how large a podcast’s potential audience is, in order to better quantify “how underserved” a given podcast is and how much success we should expect when recommending it. This will help us to focus our limited recommendation budget on the podcasts that can benefit the most from it.
More details about this work can be found in our paper: Leveraging Semantic Information to Facilitate the Discovery of Underserved Podcasts.Maryam Aziz, Alice Wang, Aasish Pappu, Hugues Bouchard,Yu Zhao, Benjamin Carterette and Mounia Lalmas CIKM 2021